The promise of AI for addressing pressing drug discovery inefficiencies, according to large pharma experts
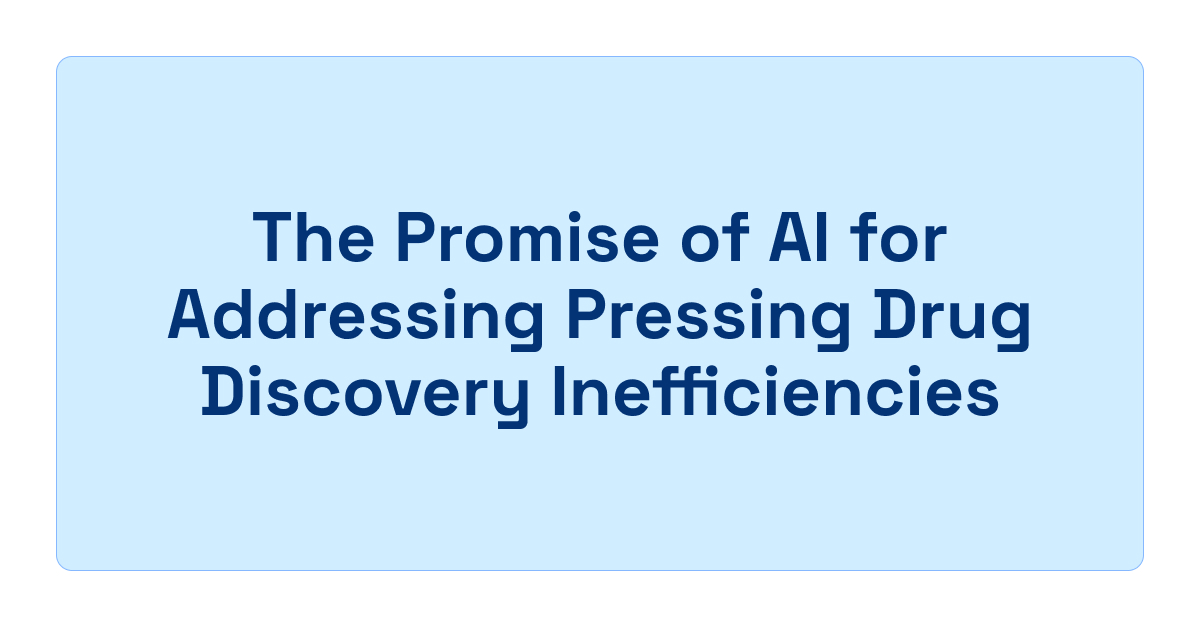
What is holding back drug discovery efficiency?
At the recent Festival of Genomics conference, Sergei Yakneen (Isomorphic Labs) asserted that drug discovery efficiency has been in an exponential decline. There are multiple bottlenecks in the process, including a limited understanding of disease biology, difficulty in ascertaining compound quality, and a lack of scalability - success for one biological target does not usually generalise to the next target or indication. In this blog post, we dive into the insights shared by pharma experts on the promise AI holds for addressing these challenges.
The Artificial Intelligence (AI) promise for drug discovery efficiency
Providing an informative and collaborative means of accessing and analysing data by incorporating AI tools into our everyday workflows promises to improve efficiency within pharma companies across “user productivity, process optimisation and business innovation” (Elif Ozkirimli, Roche). The full impact of such tools, however, is difficult to accurately measure. Anecdotally, what have we learned so far?
AI can be used to advance science and improve productivity in drug discovery in numerous ways: redefining wet lab approaches, optimising clinical trials, and enabling R&D with hub services and capabilities (Tom Diethe, AstraZeneca).
Victor Neduva (Roche) nicely summarised some key findings of strategies that have demonstrated improvements so far, including the use of API driven integration into other applications, simplifying and automating data ingestion when possible, and ensuring we produce “FAIR enough” data. There are, however, key areas where the fruits of AI labour still need improvement; the ability to extract actionable knowledge, democratising access to expert scientific perspectives, and providing ready access to the underpinning evidence as soon as the data becomes available. Without improvements in these areas, significant increases in R&D productivity will be unlikely. However, there is a balance to strike as “the relentless drive to improve productivity and efficiency will lead to more failures later down the line” (Toby Johnson, GSK).
How then, do we encourage the scientific community to trust and use AI models?
The answer lies in using as collaborative an approach as possible. Krishna Bulusu (AstraZeneca) emphasised the need to work with the subject matter experts throughout development, rather than using AI to create predictions that are then passed to the bench scientists to test. We need a standard conception of what “good” and “useful” looks like - if there is evidence that AI models are capable of producing results that users would expect, this could instill more confidence in the more novel data produced (Kelly Zalocusky, Recursion). Or perhaps we need a complete shift in our mindset; “we are all trying to make AI think like us; maybe if we stop doing this we’ll find some really useful novel information” - Krishna Bulusu (AstraZeneca).
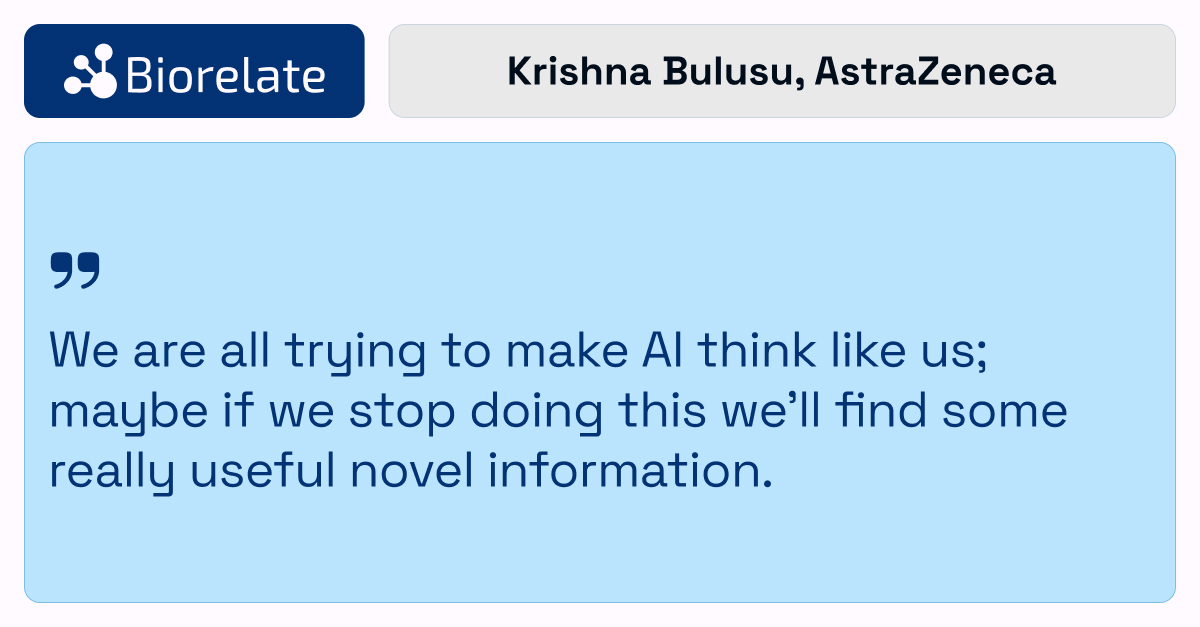
At Biorelate, we help pharma get maximum value from advanced Artificial Intelligence technologies by curating the highest-quality data from unstructured sources (literature, patents, etc.), providing the critical context needed to train AI models effectively. Our causal models embed explainable, mechanistic biology—ensuring AI delivers real impact in drug discovery programs. Start a conversation with us about accelerating your drug discovery programmes with higher quality, more explainable data by contacting us at info@biorelate.com and explore more at www.biorelate.com
Latest News
Discover new insights and updates for data science in biopharma