The importance of data quality in 2025, according to pharma leaders
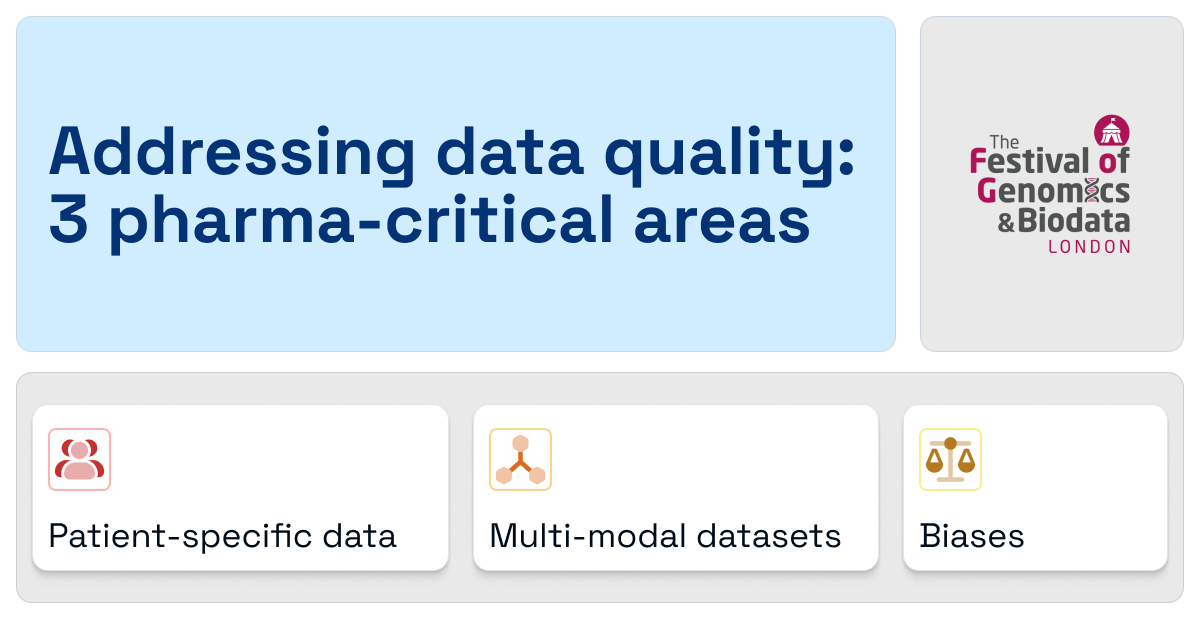
To produce high quality data, AI models need to be trained on high quality data (the "garbage in, garbage out" issue). Data “quality” can have many meanings depending on the specific aspect of data being discussed. As Brendan Frey (Deep Genomics) stated, AI has not yet met up to our expectations; 10 years of “narrow AI” has resulted in the failure to achieve “drug discovery intelligence”. Specialised AI models tend to be trained on specific datasets without considering a broader range of data sources or types within the benchmark, leading to incomplete or skewed outputs.
Here I will address three key areas of data quality in AI considered as important by pharma leaders at the recent Festival of Genomics conference: biases in data, incorporation of multimodal datasets and availability of patient-specific data.
Biased benchmark data
Data produced from scientific research is historically biased, not only in systematic errors that can occur during experimental design and execution, but also in the demographics of patient samples used (for example gender bias or a lack of data for certain ethnic minorities). This causes a significant issue for the drug development process, as new treatments will inherently be catered towards those who fit into the favoured demographic. This issue is further amplified when the role of AI in drug discovery and development is considered, as “using historic data for benchmarking creates a big bias” (Toby Johnson, GSK). Are we training inherent bias into our models and how do we mitigate this?
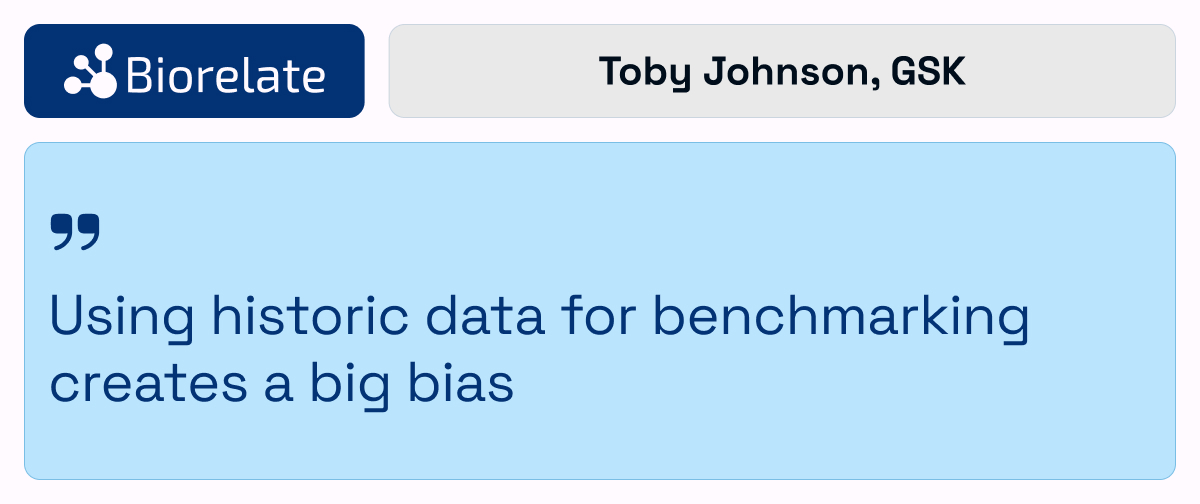
As summarised by Shruti Shikhare (AstraZeneca), the use of knowledge graphs in target discovery have demonstrated some tangible successes; knowledge graphs can create explainability where data is disparate. If we include multimodal patient data, algorithms can be developed for patient stratification. However, access to much more patient data is needed to reduce the inherent bias, and it needs to be “FAIR, diverse and standardised”.
If sufficient, diverse data isn’t available, can we produce it artificially? As discussed by Helena Andres-Terre (UCB Pharma) synthetic data (data that is created to be intentional and task-specific, designed to meet a particular analytical need) could be the answer. However, producing such data still requires good quality underlying data. Furthermore, there is no universal metric to assess the quality of synthetic data; how do we ensure there is no model drift due to biases in the training data? Patient confidentiality is also an important factor that must be considered. A careful tradeoff between data utility, fidelity and privacy must be reached for synthetic data to be a truly useful resource in contributing to reducing the bias in biological data.
Multimodal data
Another aspect that is crucial in ensuring AI models produce high quality data is the inclusion of multiple different types of data into the model. Currently, multimodal data is being leveraged within pharma companies in a number of ways, including assessing sub-groups of the population that will benefit the most from disease prevention measures (Toby Johnson, GSK). However, as summarised by Victor Neduva (Roche), whilst multimodal data can be leveraged to accelerate drug discovery, all data types must be “minable and aligned” to ensure their incorporation into training models.
Multiple pharma leaders commented that we need “real world evidence” to make robust associations within datasets, but navigating the noisy space is complicated. Zhihao Ding (Boehringer Ingelheim) commented that there are exciting opportunities to look into the underlying raw data behind studies, as well as molecular data such as large proteomics datasets and longitudinal profiling. There is also a wealth of data collected in clinical trials that is not used in study reports and therefore is not readily available for mining, due to it not directly relating to treatments or cures in question. Without access to such resources we may be missing crucial information that could aid in other areas of biomedical research (Toby Johnson, GSK).
Whilst there is certainly work to be done to have the right data available, access to samples has improved in recent years. With this increased availability comes more opportunities within pharma, including identifying new modalities; small molecules, antibodies, oligos, ADCs and identifying effective target combinations. Improvements in data availability become particularly pertinent when considering that pharma companies have reached a bifurcation in the field - it is becoming increasingly harder to develop new medicines, with increasing competition within the drug discovery space. Should the focus now be on trying to find a “mega blockbuster” drug, or narrower niches of personalised medicine where success is more likely but only a select few patients a year will benefit? (Toby Johnson, GSK).
Personalised patient data
Having a more well-rounded sample of data, particularly more real word evidence to train AI models can help with identifying the right targets for the right patients and create more personalised treatment plans. Zhihao Ding (Boehringer Ingelheim) summarised three main causal human biology data principles that must be considered to better represent patients digitally; genetics (specifically, how genetics impact biological functions), multimodal data and longitudinal data. These data then need to be integrated across multiple sites to allow accessibility and collaboration by all relevant research departments (Victor Neduva, Roche).
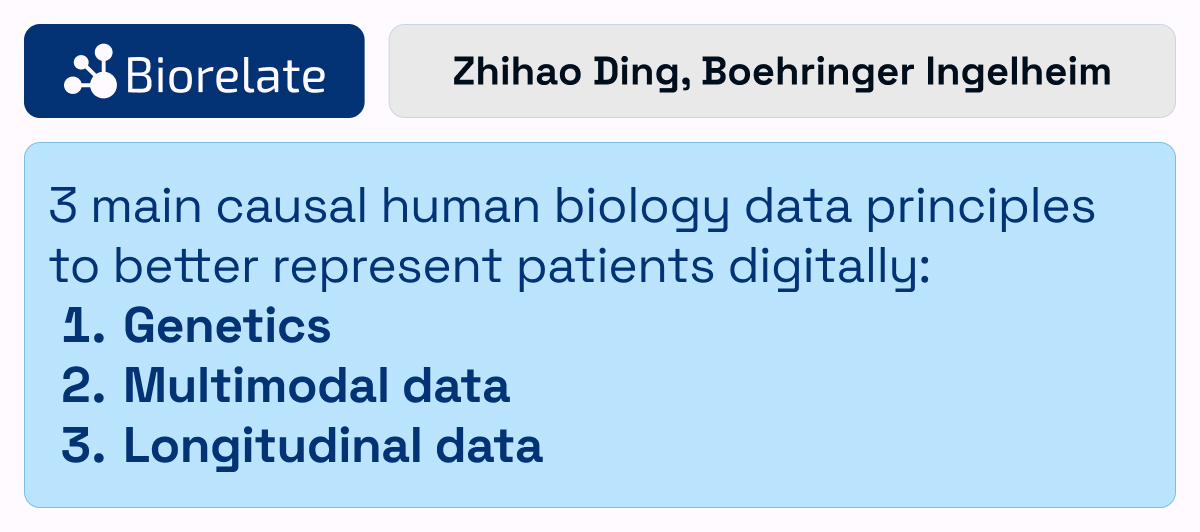
Having access to real world evidence doesn’t just affect the drug development pipeline, but also in prescribing drugs. Recent AI tools now promise to help healthcare professionals prescribe the correct drugs for patients, aiming to reduce safety concerns or human error. For this to work effectively requires a good understanding of the underlying disease and available medicines, as well as access to relevant data, sufficient for assessment of individual patients. This data is not always readily available and algorithmic bias in AI models further adds to the complexity of this process (Harriet Dickinson, Gilead Sciences).
The question still remains; how can we easily analyse large data, combining large cohorts with smaller, less represented sample sizes? Deep learning models can be used to convert different data types into a digestible understanding of biology (Zhihao Ding, Boehringer Ingelheim) but the resulting output has to be “meaningful for people who are developing drugs” (Brendan Frey, Deep Genomics).
At Biorelate, we help pharma get maximum value from advanced Artificial Intelligence technologies by curating the highest-quality data from unstructured sources (literature, patents, etc.), providing the critical context needed to train AI models effectively. Our causal models embed explainable, mechanistic biology—ensuring AI delivers real impact in drug discovery programs. Start a conversation with us about accelerating your drug discovery programmes with higher quality, more explainable data by contacting us at info@biorelate.com and explore more at www.biorelate.com
Latest News
Discover new insights and updates for data science in biopharma